class: center, middle, inverse, title-slide # Module 3: Medicare Advantage Quality and Regression Discontinuity ## Part 3: RD in Practice ### Ian McCarthy | Emory University ### Econ 470 & HLTH 470 --- <!-- Adjust some CSS code for font size and maintain R code font size --> <style type="text/css"> .remark-slide-content { font-size: 30px; padding: 1em 2em 1em 2em; } .remark-code { font-size: 15px; } .remark-inline-code { font-size: 20px; } </style> <!-- Set R options for how code chunks are displayed and load packages --> # MA Data ```r ma.data <- read_rds(here("data/final_ma_data.rds")) ``` ```r ma.data.clean <- ma.data %>% * filter(!is.na(avg_enrollment) & year==2009 & !is.na(partc_score)) ``` --- # Calculate raw average rating ```r ma.data.clean <- ma.data.clean %>% mutate(raw_rating=rowMeans( cbind(breastcancer_screen,rectalcancer_screen,cv_cholscreen,diabetes_cholscreen, glaucoma_test,monitoring,flu_vaccine,pn_vaccine,physical_health, mental_health,osteo_test,physical_monitor,primaryaccess, hospital_followup,depression_followup,nodelays,carequickly, overallrating_care,overallrating_plan,calltime, doctor_communicate,customer_service,osteo_manage, diabetes_eye,diabetes_kidney,diabetes_bloodsugar, diabetes_chol,antidepressant,bloodpressure,ra_manage, copd_test,betablocker,bladder,falling,appeals_timely, appeals_review), na.rm=T)) %>% select(contractid, planid, fips, avg_enrollment, first_enrollment, last_enrollment, state, county, raw_rating, partc_score, avg_eligibles, avg_enrolled, premium_partc, risk_ab, Star_Rating, bid, avg_ffscost, ma_rate) ``` --- # Distribution of star ratings ```r ma.data.clean %>% ggplot(aes(x=as.factor(Star_Rating))) + geom_bar() + labs( x="Star Rating", y="Count of Plans", title="Frequency Distribution of Star Ratings" ) + theme_bw() ``` .plot-callout[ <img src="03-ma-quality3_files/figure-html/rating-count-callout-1.png" style="display: block; margin: auto;" /> ] --- # Distribution of star ratings <img src="03-ma-quality3_files/figure-html/rating-count-output-1.png" style="display: block; margin: auto;" /> --- # Enrollments and star ratings ``` ## ## Call: ## lm(formula = avg_enrollment ~ factor(Star_Rating), data = ma.data.clean) ## ## Residuals: ## Min 1Q Median 3Q Max ## -627 -388 -214 -51 41908 ## ## Coefficients: ## Estimate Std. Error t value Pr(>|t|) ## (Intercept) 87.31 43.32 2.016 0.04387 * ## factor(Star_Rating)2 32.75 50.62 0.647 0.51758 ## factor(Star_Rating)2.5 194.65 47.15 4.128 3.67e-05 *** ## factor(Star_Rating)3 433.95 49.84 8.707 < 2e-16 *** ## factor(Star_Rating)3.5 470.91 53.47 8.808 < 2e-16 *** ## factor(Star_Rating)4 552.30 57.91 9.538 < 2e-16 *** ## factor(Star_Rating)4.5 272.36 82.68 3.294 0.00099 *** ## --- ## Signif. codes: 0 '***' 0.001 '**' 0.01 '*' 0.05 '.' 0.1 ' ' 1 ## ## Residual standard error: 1440 on 17451 degrees of freedom ## Multiple R-squared: 0.01559, Adjusted R-squared: 0.01526 ## F-statistic: 46.07 on 6 and 17451 DF, p-value: < 2.2e-16 ``` --- # Problems - Certainly not the effect of a higher rating... - Lots of things unobserved, like - actual quality - perceived quality - prices --- # Effect of 3-star rating .left-code[ ```r ma.rd1 <- ma.data.clean %>% filter(Star_Rating==2 | Star_Rating==2.5) ``` ] .right-plot[ ```r ma.rd1 %>% ggplot(aes(x=raw_rating)) + geom_bar(width=.025) + theme_bw() + labs( x="Running Variable", y="Number of Plans", title="Distribution of Raw Scores" ) ``` <img src="03-ma-quality3_files/figure-html/unnamed-chunk-6-1.png" style="display: block; margin: auto;" /> ] --- # Note about scores CMS does more than just an average... - variance across individual metrics - high variance is punished, low variance rewarded --- # RD estimates ```r ma.rd1 <- ma.rd1 %>% mutate(score = raw_rating - 2.25, treat = (score>=0), window1 = (score>=-.175 & score<=.175), window2 = (score>=-.125 & score<=.125), mkt_share = avg_enrollment/avg_eligibles, ln_share = log(mkt_share), score_treat=score*treat) star25.1 <- lm(mkt_share ~ score + treat, data=ma.rd1) star25.2 <- lm(mkt_share ~ score + treat, data= (ma.rd1 %>% filter(window1==TRUE))) star25.3 <- lm(mkt_share ~ score + treat + score_treat, data= (ma.rd1 %>% filter(window1==TRUE))) star25.4 <- lm(mkt_share ~ score + treat + score_treat, data= (ma.rd1 %>% filter(window2==TRUE))) est1 <- as.numeric(star25.1$coef[3]) est2 <- as.numeric(star25.2$coef[3]) est3 <- as.numeric(star25.3$coef[3]) est4 <- as.numeric(star25.4$coef[3]) ``` --- # RD estimates <table style="text-align:center"><tr><td colspan="5" style="border-bottom: 1px solid black"></td></tr><tr><td style="text-align:left"></td><td colspan="4">mkt_share</td></tr> <tr><td style="text-align:left"></td><td>(1)</td><td>(2)</td><td>(3)</td><td>(4)</td></tr> <tr><td colspan="5" style="border-bottom: 1px solid black"></td></tr><tr><td style="text-align:left">Raw Score</td><td>0.030<sup>***</sup></td><td>-0.044<sup>***</sup></td><td>-0.066<sup>***</sup></td><td>-0.085<sup>***</sup></td></tr> <tr><td style="text-align:left"></td><td>(0.002)</td><td>(0.009)</td><td>(0.012)</td><td>(0.013)</td></tr> <tr><td style="text-align:left">Treatment</td><td>-0.008<sup>***</sup></td><td>0.009<sup>***</sup></td><td>0.008<sup>***</sup></td><td>0.006<sup>***</sup></td></tr> <tr><td style="text-align:left"></td><td>(0.001)</td><td>(0.002)</td><td>(0.002)</td><td>(0.002)</td></tr> <tr><td style="text-align:left">Score x Treat</td><td></td><td></td><td>0.049<sup>***</sup></td><td>0.125<sup>***</sup></td></tr> <tr><td style="text-align:left"></td><td></td><td></td><td>(0.019)</td><td>(0.026)</td></tr> <tr><td colspan="5" style="border-bottom: 1px solid black"></td></tr><tr><td style="text-align:left">Bandwith</td><td>0.5</td><td>0.175</td><td>0.175.</td><td>0.125</td></tr> <tr><td style="text-align:left">Observations</td><td>9,006</td><td>3,095</td><td>3,095</td><td>2,962</td></tr> <tr><td colspan="5" style="border-bottom: 1px solid black"></td></tr><tr><td style="text-align:left"><em>Note:</em></td><td colspan="4" style="text-align:right"><sup>*</sup>p<0.1; <sup>**</sup>p<0.05; <sup>***</sup>p<0.01</td></tr> </table> --- # Interpretation - OLS on full sample: -0.8% increase in market shares among 2.5-star plans versus 2-star plan - RD on 0.175 bandwidth: 0.9% increase when imposing constant slopes, 0.8% increase when allowing for differential slopes - RD on 0.125 bandwidth: 0.6% increase (again allowing for differential slopes) --- # Built-in RD packages ```r library(rdrobust) rdplot(y=ma.rd1$mkt_share, x=ma.rd1$score, binselect="es", title="RD Plot: Market Share", x.label="Summary Score", y.label="Market Share", masspoints="off") ``` .plot-callout[ <img src="03-ma-quality3_files/figure-html/rd-ma-plot-callout-1.png" style="display: block; margin: auto;" /> ] --- # RD Plot <img src="03-ma-quality3_files/figure-html/rd-ma-plot-output-1.png" style="display: block; margin: auto;" /> --- # Estimates from RD package ```r est1 <- rdrobust(y=ma.rd1$mkt_share, x=ma.rd1$score, c=0, h=0.125, p=1, kernel="uniform", vce="hc0", masspoints="off") ``` --- # Estimates from RD package ``` ## Call: rdrobust ## ## Number of Obs. 9006 ## BW type Manual ## Kernel Uniform ## VCE method HC0 ## ## Number of Obs. 3024 5982 ## Eff. Number of Obs. 2702 260 ## Order est. (p) 1 1 ## Order bias (q) 2 2 ## BW est. (h) 0.125 0.125 ## BW bias (b) 0.125 0.125 ## rho (h/b) 1.000 1.000 ## ## ============================================================================= ## Method Coef. Std. Err. z P>|z| [ 95% C.I. ] ## ============================================================================= ## Conventional 0.006 0.003 2.034 0.042 [0.000 , 0.012] ## Robust - - 5.870 0.000 [0.024 , 0.049] ## ============================================================================= ``` --- # Optimal bandwidth ```r estopt <- rdrobust(y=ma.rd1$mkt_share, x=ma.rd1$score, c=0, p=1, kernel="uniform", vce="hc0", masspoints="off") ``` --- # Estimates with optimal bandwidth ``` ## Call: rdrobust ## ## Number of Obs. 9006 ## BW type mserd ## Kernel Uniform ## VCE method HC0 ## ## Number of Obs. 3024 5982 ## Eff. Number of Obs. 234 91 ## Order est. (p) 1 1 ## Order bias (q) 2 2 ## BW est. (h) 0.054 0.054 ## BW bias (b) 0.123 0.123 ## rho (h/b) 0.442 0.442 ## ## ============================================================================= ## Method Coef. Std. Err. z P>|z| [ 95% C.I. ] ## ============================================================================= ## Conventional 0.034 0.008 4.556 0.000 [0.020 , 0.049] ## Robust - - 4.335 0.000 [0.018 , 0.049] ## ============================================================================= ``` --- # Estimates for other rating thresholds ```r summary(est225) ``` ``` ## Call: rdrobust ## ## Number of Obs. 9006 ## BW type Manual ## Kernel Uniform ## VCE method HC0 ## ## Number of Obs. 3024 5982 ## Eff. Number of Obs. 2702 260 ## Order est. (p) 1 1 ## Order bias (q) 2 2 ## BW est. (h) 0.125 0.125 ## BW bias (b) 0.125 0.125 ## rho (h/b) 1.000 1.000 ## ## ============================================================================= ## Method Coef. Std. Err. z P>|z| [ 95% C.I. ] ## ============================================================================= ## Conventional 0.006 0.003 2.034 0.042 [0.000 , 0.012] ## Robust - - 5.870 0.000 [0.024 , 0.049] ## ============================================================================= ``` --- # Estimates for other rating thresholds ```r summary(est275) ``` ``` ## Call: rdrobust ## ## Number of Obs. 9396 ## BW type Manual ## Kernel Uniform ## VCE method HC0 ## ## Number of Obs. 5982 3414 ## Eff. Number of Obs. 243 1502 ## Order est. (p) 1 1 ## Order bias (q) 2 2 ## BW est. (h) 0.125 0.125 ## BW bias (b) 0.125 0.125 ## rho (h/b) 1.000 1.000 ## ## ============================================================================= ## Method Coef. Std. Err. z P>|z| [ 95% C.I. ] ## ============================================================================= ## Conventional 0.021 0.004 4.700 0.000 [0.012 , 0.029] ## Robust - - 2.218 0.027 [0.003 , 0.050] ## ============================================================================= ``` --- # Estimates for other rating thresholds ```r summary(est325) ``` ``` ## Call: rdrobust ## ## Number of Obs. 5525 ## BW type Manual ## Kernel Uniform ## VCE method HC0 ## ## Number of Obs. 3826 1699 ## Eff. Number of Obs. 888 629 ## Order est. (p) 1 1 ## Order bias (q) 2 2 ## BW est. (h) 0.125 0.125 ## BW bias (b) 0.125 0.125 ## rho (h/b) 1.000 1.000 ## ## ============================================================================= ## Method Coef. Std. Err. z P>|z| [ 95% C.I. ] ## ============================================================================= ## Conventional 0.016 0.004 4.568 0.000 [0.009 , 0.024] ## Robust - - 7.504 0.000 [0.025 , 0.043] ## ============================================================================= ``` --- # Estimates for other rating thresholds ```r summary(est375) ``` ``` ## Call: rdrobust ## ## Number of Obs. 3515 ## BW type Manual ## Kernel Uniform ## VCE method HC0 ## ## Number of Obs. 2625 890 ## Eff. Number of Obs. 628 619 ## Order est. (p) 1 1 ## Order bias (q) 2 2 ## BW est. (h) 0.125 0.125 ## BW bias (b) 0.125 0.125 ## rho (h/b) 1.000 1.000 ## ## ============================================================================= ## Method Coef. Std. Err. z P>|z| [ 95% C.I. ] ## ============================================================================= ## Conventional -0.004 0.003 -1.705 0.088 [-0.010 , 0.001] ## Robust - - -2.726 0.006 [-0.022 , -0.004] ## ============================================================================= ``` --- # Manipulation of the running variable .center[ 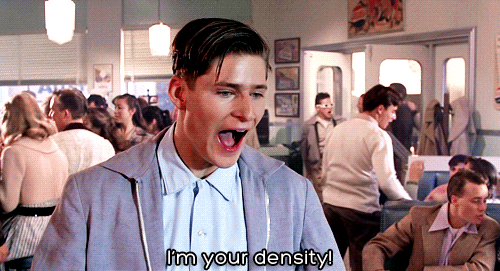 ] --- # Manipulation of the running variable ```r dens225 <- rddensity(ma.rd225$score, c=0) rdplotdensity(dens225, ma.rd225$score) ``` <img src="03-ma-quality3_files/figure-html/unnamed-chunk-18-1.png" style="display: block; margin: auto;" /> ``` ## $Estl ## Call: lpdensity ## ## Sample size 3032 ## Polynomial order for point estimation (p=) 2 ## Order of derivative estimated (v=) 1 ## Polynomial order for confidence interval (q=) 3 ## Kernel function triangular ## Scaling factor 0.33659078289839 ## Bandwidth method user provided ## ## Use summary(...) to show estimates. ## ## $Estr ## Call: lpdensity ## ## Sample size 5982 ## Polynomial order for point estimation (p=) 2 ## Order of derivative estimated (v=) 1 ## Polynomial order for confidence interval (q=) 3 ## Kernel function triangular ## Scaling factor 0.664186563020544 ## Bandwidth method user provided ## ## Use summary(...) to show estimates. ## ## $Estplot ``` <img src="03-ma-quality3_files/figure-html/unnamed-chunk-18-2.png" style="display: block; margin: auto;" /> --- # Manipulation of the running variable ```r summary(dens275) ``` ``` ## ## Manipulation testing using local polynomial density estimation. ## ## Number of obs = 9396 ## Model = unrestricted ## Kernel = triangular ## BW method = estimated ## VCE method = jackknife ## ## c = 0 Left of c Right of c ## Number of obs 5982 3414 ## Eff. Number of obs 4113 2075 ## Order est. (p) 2 2 ## Order bias (q) 3 3 ## BW est. (h) 0.228 0.228 ## ## Method T P > |T| ## Robust 33.7033 0 ## ## ## P-values of binomial tests (H0: p=0.5). ## ## Window Length / 2 <c >=c P>|T| ## 0.017 0 27 0.0000 ## 0.033 2 125 0.0000 ## 0.050 57 125 0.0000 ## 0.067 94 248 0.0000 ## 0.083 94 302 0.0000 ## 0.100 167 515 0.0000 ## 0.117 233 1499 0.0000 ## 0.133 243 1502 0.0000 ## 0.150 3471 1798 0.0000 ## 0.167 3471 1826 0.0000 ``` --- # Covariate balance ```r match.dat <- matchit(treat~premium_partc + ma_rate, data=ma.rd225 %>% filter(window2==TRUE, !is.na(treat), !is.na(premium_partc), !is.na(ma_rate)), method=NULL, distance="mahalanobis") ``` --- # Covariate balance <img src="03-ma-quality3_files/figure-html/unnamed-chunk-21-1.png" style="display: block; margin: auto;" /> --- # RD with discrete variables - Allow for fewer mass points - Assume random assignment between mass points - Inference using Fisher's exact test